AI Transformation
5 Steps for a Successful AI Transformation
“We’ve arranged a civilization in which most crucial elements profoundly depend on science and technology. We have also arranged things so that almost no one understands science and technology.” …
Carl Sagan
Artificial Intelligence (AI) is making technology a lot more accessible, creating new opportunities, and challenging businesses to adapt or risk being left behind. As AI innovation accelerates, companies that fail to integrate AI into their core operations may lose their competitive edge. Here is an outline of the five critical steps for a successful AI transformation, ensuring that businesses can harness AI effectively and avoid common pitfalls.
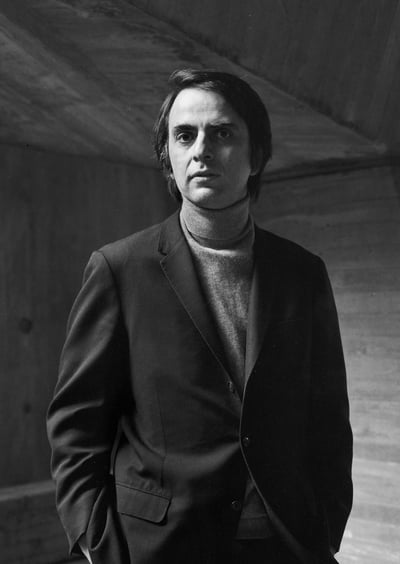
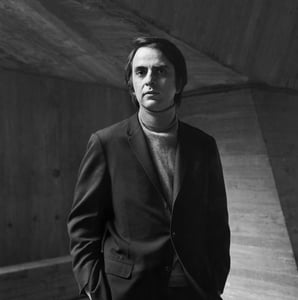
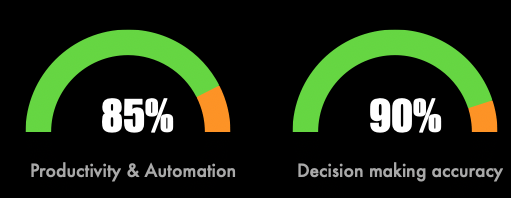
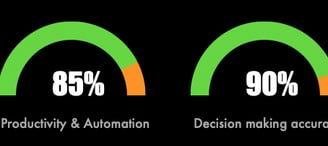
Tangible benefits
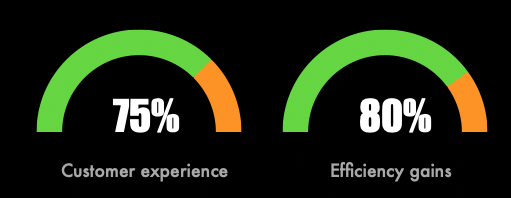
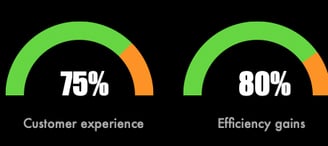
AI own assessment of its benefits
Why it matters
The window of opportunity is moving quickly
AI is not just a technological shift; it is a business transformation that affects competition, user expectations, and industry standards. Companies that fail to act now risk obsolescence. Here’s why AI transformation is crucial:
Fierce Competition
Companies across sectors are already leveraging AI to gain a strategic edge by optimizing operations, predicting market shifts, and refining customer interactions. As these AI-enabled organizations become more efficient, their ability to outpace competitors grows exponentially. Organizations that fail to incorporate AI risk being outmaneuvered by data-driven rivals and finding themselves locked out of profitable market segments.
Rising User Expectations
Today’s consumers and corporate clients alike demand instantaneous, highly personalized experiences. AI-powered platforms can analyze behavior patterns in real time, tailor recommendations, and offer proactive customer service. This creates a new standard for speed and personalization: businesses that cannot keep pace will struggle to maintain relevance and loyalty among increasingly tech-savvy audiences.
Rapid Innovation
AI-driven processes shorten development cycles, turning months of research and prototyping into weeks—or even days—by automating tasks, generating insights, and discovering patterns with remarkable speed. This disruptive level of innovation forces businesses to adapt quickly, or risk releasing outdated products and services. To thrive, organizations must keep pace with this accelerated environment by integrating AI at the core of their innovation strategy.
Lower Barriers to Entry
Traditional market advantages, such as large capital reserves or extensive physical infrastructure, can be rapidly undermined by data-smart upstarts utilizing AI. By automating key processes and leveraging open-source AI technologies, newer entrants can operate with greater agility and lower costs. Established players that hesitate to adopt AI run the risk of being overtaken by these lean, tech-driven challengers.
Massive Value Transfers
AI will drive significant economic shifts. Organizations that fully embrace AI-driven transformation will capture new revenue streams, enhance their value propositions, and reconfigure the competitive landscape. However, businesses that allow external partners, vendors, or competitors to spearhead AI adoption may lose control over critical value-added functions. If left unchecked, this can lead to lost revenue, compromised market share, and a diminished role within the broader ecosystem.
By viewing AI as a holistic business transformation—rather than a narrow technology project—leaders can position their organizations to not only survive but actively shape the competitive market of the future.
Why AI Transformation Fails: Common Mistakes
Despite its potential, many AI transformation initiatives fail due to poor execution.
Speed of Execution Is Not the Full Story
AI can generate outputs at an unprecedented pace—reports, product features, or customer insights. However, raw output alone does not automatically translate to effective decision-making or superior customer experiences. Organizations need to measure how AI outcomes advance strategic goals and create real value.
Strategic Alignment and Product Fit
AI should enhance, not complicate, your product or service offerings. When teams are purely output-driven, they risk rolling out AI features that aren’t aligned with market needs or confuse customers. Ongoing collaboration between product managers, UX designers, and AI specialists is vital to ensuring AI outputs are integrated in a way that resonates with end users and supports the broader roadmap.
Operational Disruptions
Uncontrolled AI-driven changes can disrupt existing workflows and production systems. Proper change management—engaging stakeholders, offering training, and rolling out solutions incrementally—helps minimize downtime or misalignment. Remember, AI is an evolving technology that requires continuous feedback loops and iteration to deliver sustained benefits.
Inability to accelerate Decision-Making
In many cases, the greatest value of AI lies in quicker, more accurate decision-making. However, simply having AI-generated insights is not enough; a robust decision-making framework is needed to interpret recommendations, weigh risks, and take measured actions. Without the right processes in place, organizations may overlook valuable insights or make misguided choices.
Recommendation: Use AI to augment, not replace, strategic thinking. Focus on how AI supports key business objectives and delivers meaningful, user-centric experiences. Implement robust governance, clear communication, and continuous feedback mechanisms to ensure AI’s pace of execution aligns seamlessly with your broader vision.
Step 0: Agility as a precursor to Artificial Intelligence
Agility is embedded is the pure essence of AI (trial and error)
Agile methodology plays a crucial role in preparing organizations for AI transformation by fostering a culture of adaptability, continuous learning, and iterative development. Agile’s iterative approach aligns with AI model development, as AI solutions require frequent testing, refining, and retraining based on new data and insights. Agile’s emphasis on sprints, iterations, and continuous feedback makes it an ideal methodology for AI projects. Just like Agile teams iterate on software features, AI teams iterate on models by improving data quality, tuning algorithms, and optimizing performance.
Cross-functional collaboration enables AI readiness. Agile promotes cross-functional teams, which is essential for AI, as it requires collaboration between data scientists, engineers, business leaders, and end-users. Agile frameworks like Scrum and SAFe facilitate structured collaboration, ensuring AI initiatives align with business goals. AI success depends on integrating domain expertise with technical capabilities, and Agile creates an environment where teams communicate effectively.
Data-driven decision-making becomes a habit in an Agile environment. AI thrives on data, and Agile encourages teams to adopt a mindset of data-driven experimentation. Agile teams continuously measure outcomes using KPIs, metrics, and analytics, fostering a culture that naturally transitions into AI-driven insights. Organizations used to making iterative, data-backed decisions in Agile will find it easier to trust AI-generated recommendations.
Agile’s flexibility helps overcome AI uncertainty. AI projects often face uncertainty in data quality, algorithm performance, and business impact. Agile helps teams respond to change rather than following rigid long-term plans, making it easier to pivot when AI models need adjustments. Companies comfortable with Agile will find it easier to experiment with AI use cases and scale successful initiatives.
AI automation enhances Agile efficiency. AI can automate repetitive Agile processes, such as predictive analytics for backlog prioritization, automated testing and defect detection in software development, and AI-driven chatbots for managing Agile workflows. Organizations already practicing Agile are well-positioned to integrate AI tools to improve efficiency and increase team productivity.
Agile’s customer-centric approach aligns with AI personalization. AI enables hyper-personalized user experiences, but Agile teams are already trained to iterate based on user feedback. Agile’s focus on MVPs (Minimum Viable Products) and rapid deployment aligns with how AI models require constant A/B testing and validation. By maintaining Agile’s customer-first mindset, companies ensure that AI delivers real value instead of just technological novelty.
Agile governance lays the groundwork for AI ethics and compliance. AI raises concerns around bias, transparency, and accountability. Agile governance models, such as Lean Portfolio Management, help ensure that AI initiatives align with organizational goals, ethical standards, and compliance regulations. Agile’s retrospectives and continuous improvement mindset support the ongoing monitoring and adjustment of AI systems.
Agile methodology cultivates the mindset, practices, and processes needed for AI success. By embracing Agile, organizations develop a culture of iteration and learning, build cross-functional teams for AI collaboration, use data-driven decision-making, enhance adaptability to rapid technological changes, and improve customer-centricity, which AI enhances through personalization. Companies that successfully implement Agile methodologies are in a stronger position to leverage AI for competitive advantage and digital transformation.
Agile Coaches are ideal for facilitating AI adoption because they drive cultural transformation, foster collaboration, and promote iterative learning. Their expertise in change management helps teams navigate AI’s uncertainty, align initiatives with business goals, and adopt data-driven decision-making. By encouraging experimentation, ethical AI practices, and continuous improvement, Agile Coaches ensure organizations successfully integrate AI into their workflows.
Five Steps for a Successful AI Transformation
“The price of inaction is far greater than the cost of making a mistake.”
Meister Eckhar
Step 1: Cultivate a Strong AI Culture (Leadership alignment)
A robust AI transformation begins with a cultural shift at all levels of the organization. Leadership must take an active role in fostering an AI-driven mindset, ensuring alignment across teams, and addressing potential resistance.
Addressing employees’ concerns is crucial as AI adoption often triggers uncertainty, particularly around job security and shifting responsibilities. Transparent communication from leadership is essential. Clearly articulate AI’s role as an enabler of efficiency, not a replacement for human talent. Reinforce the message that AI is designed to augment decision-making, automate repetitive tasks, and create new growth opportunities rather than eliminate jobs.
Reinforcing the core team is essential for AI transformation. It should be driven by a cross-functional team that understands both business strategy and customer needs. This team should include business leaders, AI specialists, data scientists, and operational managers who can align AI initiatives with corporate objectives. Providing them with the right resources and executive support ensures a structured and strategic approach to AI implementation.
Encouraging innovation is key to fostering a culture of experimentation by rewarding employees who identify AI-driven efficiencies or propose innovative AI applications. Implementing a structured feedback mechanism to capture insights from frontline employees who engage with AI systems daily ensures that the organization benefits from practical, on-the-ground perspectives. Recognizing and incentivizing AI adoption will accelerate organizational buy-in and integration.
Promoting AI champions within the organization will help drive transformation. Identifying and empowering employees who are enthusiastic about AI and have a deep understanding of how it can drive value is essential. These AI champions should act as internal advocates, mentoring their peers and facilitating AI literacy across teams. Establishing a network of AI ambassadors within the company ensures that AI transformation is embraced organically rather than perceived as a top-down mandate.
Step 2: Take Control of Partners & MSP Relationships
AI transformation does not occur in isolation; it requires a collaborative approach with third-party vendors, managed service providers (MSPs), and technology partners. Organizations must proactively manage these relationships to ensure AI adoption aligns with business objectives and regulatory requirements.
Reviewing existing contracts is an essential first step. Conducting a thorough evaluation of current agreements with vendors and MSPs helps identify AI-related clauses, including ownership of AI-generated data, intellectual property rights, and service-level expectations. Ensuring that AI integration benefits both the organization and its partners while maintaining transparency and compliance with evolving regulatory standards is critical.
Mandatory AI transparency should be required from vendors and partners, ensuring they disclose their use of AI in service delivery, data processing, and decision-making. This ensures alignment with the organization’s ethical AI policies, mitigates risks associated with black-box AI models, and fosters a collaborative approach to AI governance. Establishing clear accountability measures to address AI-related biases, errors, and security concerns strengthens the integrity of AI implementation.
Enabling knowledge transfer between partners and internal teams ensures AI expertise does not remain siloed within vendors or external service providers. Establishing structured processes to facilitate continuous AI knowledge sharing can include co-development initiatives, training sessions, and joint AI pilot projects. This approach enhances the internal team’s AI proficiency while reducing dependency on external vendors over time.
By ensuring that AI initiatives are strategically integrated within both internal operations and external partnerships, organizations can maximize the value of AI transformation while minimizing risks and inefficiencies.
Step 3: Develop AI applications
Start Simple, Scale up...
Examples of Plug-and-Play AI Solutions:
Chatbots (Intercom, Drift)
Content Generation (Jasper, Copy.ai)
Marketing AI (Google Ads Automation)
Process Automation (Zapier, Make)
Meeting Transcription (Otter.ai, Fireflies.ai)
...
Examples of Department-Specific AI Applications:
IT & Software Development: AI-powered IT chatbots, automated code reviews, predictive maintenance.
Customer Experience: AI-driven sentiment analysis, chatbots, and personalization.
Marketing & Sales: AI-powered ads, email personalization, and automated content creation.
Operations & HR: AI for automation, workforce optimization, and compliance management.
...
Examples of Advanced AI for High-Value Impact:
AI-Powered Decision-Making - Predictive analytics for business insights
Generative AI for Innovation: Product design, software development
Hyper-automation: End-to-end business process automation
AI-Driven Cybersecurity: Real-time threat detection and fraud prevention
Supply Chain Optimization: AI-based demand forecasting and logistics
...
Step 4 - Empower decision making
AI accelerates execution, making decision-making the bottleneck.
Redefine decision levels:
Establishing clear decision-making levels is critical to ensuring efficiency, accountability, and alignment within an AI-driven organization. Business goals must be set at the executive level, where leadership defines the overarching vision, market positioning, and strategic priorities. These decisions should focus on long-term growth, competitive differentiation, and value creation, ensuring AI initiatives support broader corporate objectives.
IT strategy needs to be owned by IT leaders, who oversee architectural frameworks, platform selection, and technology governance. Their role is to ensure that AI capabilities align with business needs while maintaining scalability, security, and compliance. IT leaders must collaborate closely with other departments to prevent technology silos and ensure seamless integration of AI-driven solutions.
The product roadmap is managed by product owners and cross-functional teams who translate business objectives into actionable technology initiatives. These teams are responsible for defining feature priorities, balancing customer needs with technical feasibility, and ensuring AI-driven enhancements align with user expectations and market demands. Effective collaboration between product, engineering, and business units ensures that AI is leveraged for maximum impact.
Sprint prioritization is decided by agile teams that execute development cycles, implement AI-driven features, and iterate based on real-time feedback. Agile teams must be empowered to make day-to-day operational decisions, optimizing development speed and responsiveness. AI initiatives should be integrated into agile workflows, ensuring continuous delivery of high-value solutions that support evolving business needs.
Build Strong Cross-Functional Teams:
Successful AI transformation requires seamless collaboration between product managers, technical experts, analysts, and process owners. Each of these roles brings unique expertise to the table, and their collective efforts ensure that AI initiatives align with both strategic objectives and operational realities. Product managers provide the vision, ensuring AI solutions address real business needs and deliver value. Technical experts, including data scientists and engineers, drive the development and deployment of AI models. Analysts contribute by interpreting data insights, identifying trends, and ensuring that AI-driven decisions are based on robust analytical frameworks. Process owners bridge the gap between AI capabilities and business workflows, ensuring AI solutions integrate seamlessly into existing operations without disrupting productivity.
Encouraging autonomous decision-making within cross-functional teams enhances agility and accelerates AI adoption. Traditional hierarchical decision-making models can slow down progress, creating bottlenecks that hinder AI implementation. Instead, teams should be empowered with the authority to make tactical decisions within their scope of responsibility. Providing clear objectives, well-defined success criteria, and access to necessary resources ensures that teams can iterate quickly and adapt AI solutions in response to real-world challenges. Trusting teams to drive AI initiatives fosters innovation and accountability while reducing dependencies on top-down approvals.
Structured coordination events play a critical role in maintaining alignment across multiple teams and stakeholders. Large-scale initiatives require synchronized efforts across different functions to ensure AI projects remain on track and deliver expected outcomes. Big room planning sessions bring together all key players to align on goals, discuss interdependencies, and identify potential risks before they become roadblocks. Roadmap synchronization meetings ensure AI initiatives are integrated into the broader strategic vision, helping organizations prioritize high-impact projects while avoiding redundant efforts. These structured events create transparency, facilitate collaboration, and provide a forum for resolving challenges proactively.
By fostering strong cross-functional teams, empowering decision-making at the team level, and implementing structured coordination mechanisms, organizations can accelerate AI transformation while maintaining strategic alignment. The key to success lies in balancing autonomy with alignment, enabling teams to move quickly while ensuring their efforts contribute to the organization’s overarching AI goals.
Step 5: Measure AI Transformation Success
Rethink how you operate
Key Metrics to Track:
Reduction in dependence on MSPs & vendors is a crucial metric to determine whether AI adoption is effectively increasing internal capabilities. Organizations must assess whether AI-driven automation and decision-making are replacing previously outsourced functions, leading to reduced reliance on external managed service providers and vendors. This can be measured through a decrease in vendor-related costs, a reduction in contracted service hours, or an increase in internally managed AI workflows. A successful AI transformation should empower internal teams with the necessary skills and tools to operate independently, ensuring that external partnerships are leveraged for strategic innovation rather than operational dependencies.
Value-driven performance tracking is essential to determine whether AI implementations are delivering measurable business outcomes. Traditional performance metrics may not fully capture AI’s impact, so organizations must define key performance indicators (KPIs) that align with strategic goals. Metrics such as increased revenue, improved customer satisfaction, enhanced operational efficiency, or reduced time-to-market for new initiatives can provide tangible evidence of AI’s value. AI investments should be continuously evaluated against these KPIs to ensure they contribute to long-term business success rather than becoming isolated technological advancements without clear ROI.
Efficiency vs. actual savings must be analyzed to determine whether AI-driven productivity gains translate into tangible cost reductions. AI can significantly enhance efficiency by automating tasks, improving decision-making, and optimizing resource allocation. However, organizations must assess whether these efficiency gains lead to financial savings or merely redistribute workloads. The success of AI should be measured not only by its ability to streamline processes but also by its impact on bottom-line financials, including labor cost savings, reduced operational expenses, and improved asset utilization. If AI-driven efficiencies are not resulting in cost reductions, it may indicate a need to refine AI deployment strategies.
Innovation vs. maintenance ratio is a critical metric for evaluating whether AI initiatives are driving strategic growth rather than being consumed by routine operational maintenance. AI should be a catalyst for innovation, enabling new product development, enhancing customer experiences, and identifying new revenue streams. Organizations should assess the proportion of AI investments allocated to transformative projects versus those spent on sustaining existing processes. A high maintenance-to-innovation ratio may indicate that AI efforts are being used primarily for incremental improvements rather than for disruptive advancements that generate competitive advantage. Striking the right balance ensures that AI remains a driver of future growth rather than a tool for maintaining the status quo.
AI-first mindset adoption should be measured to determine whether AI is integrated proactively into business strategy or treated as an afterthought. A successful AI transformation requires organizations to embed AI into decision-making processes, workflows, and innovation strategies from the outset rather than applying AI reactively to existing challenges. Key indicators of an AI-first mindset include leadership commitment to AI-driven initiatives, AI literacy across teams, and the presence of AI in strategic planning discussions. Organizations should also assess how frequently AI is considered when exploring new opportunities, launching products, or optimizing operations. A reactive AI approach may lead to fragmented implementations, whereas a proactive AI strategy ensures long-term scalability and sustainable competitive advantage.
By systematically tracking these key metrics, organizations can gauge the effectiveness of their AI transformation, ensuring that AI delivers measurable business value, enhances operational efficiency, and fosters long-term innovation.
AI transformation is not just about implementing AI—it is about rethinking how businesses operate. Companies must take ownership of AI initiatives, invest in their core teams, redefine decision-making processes, and build a culture where AI is a strategic advantage rather than a disruptive force.
Organizations that follow these five steps will lead their industry’s AI revolution, rather than being left behind.
Get in touch
Transformation can lead to amazing results and
it is easy if you embrace the culture